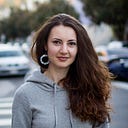

Discover more from Data Analysis Journal
A Guide to Measuring Feature Contribution to KPIs
Four Causal Analyses That Will Drive Your Next Promotion: A Guest Post by Tom Laufer, CEO & Co-founder of Loops
Welcome to my Data Analytics Journal, where I write about data science and analytics.
This month, paid subscribers learned about:
Data Analyst Toolbox: Must-have tools and applications for data analysis and data science that can save you time and effort. Sharing my personal analytics toolkit.
Methods To Accelerate A/B Testing: Strategies and statistical methods to increase test velocity. Learn what statistical methods and solutions you can leverage to speed up testing and how to balance trust and speed.
Introduction To Proxy Metrics: A guide to identifying and implementing effective proxy metrics, with examples and use cases.
Measuring the impact of product feature optimizations is not easy.
Identifying what drives top-line conversions requires significant analytical effort and time:
More feature usage does not necessarily imply higher conversion rates.
High conversion rates do not necessarily lead to improvement in retention.
Improvements in retention may not translate into increased revenue.
It all comes down to understanding the causal relationships between metrics and user actions.
To dive deeper into calculating and measuring cause-and-effect relationships, I asked Tom Laufer, CEO and Co-founder of Loops, to share with us the best practices and frameworks in analytics.
I met Tom in San Francisco a few months ago. What was intended to be a brief introduction turned into an hours-long conversation about the state of data analytics, the challenges faced by analytics teams, and ways to navigate them. It was, by far, the most refreshing, intelligent, and direct discussion on analytics I've had in a while. I knew then that I had to ask Tom to write for me, and today, I am so excited to invite him to be a guest writer.
What’s even more exciting is that I am joining Tom next week in San Francisco at the Product Analytics Leader's Meetup, where we will discuss ways to build high-impact product teams, enhance insight-actionability, avoid pitfalls in defining KPIs, introduce causal analytics, and more. Hope to see everyone there!
Tom Laufer previously led growth and analytics at Google EMEA. Over a career centered on product and growth, he has served in both product management and data analytics. In 2020, he co-founded Loops, a product analytics tool designed to help data and team maximize their KPIs by identifying hidden actionable opportunities within their product data.
Today, Tom will share his perspective on understanding cause and effect and will walk us through 4 different analyses: Conversion drivers, Retention drivers, Engagement drivers, and Feature funnel analysis.
How to Size Feature-to-KPI Contribution
As analysts, your focus is to identify what could drive your topline metrics. It’s clear you need to focus on the features, content, and journeys that drive your KPIs, but how should you do this?
The many data sources and correlations you have at hand can be very confusing. You might see that users of one feature, say “Search,” are more likely to convert. However, this does not necessarily mean you should focus on Search? While users that “Search” have some intent, this feature itself may have little impact on your other KPIs once they convert. It could be that the intent itself is the reason why these users convert or retain, and not because of the feature search. Meaning, it could be purely a correlation. That's why understanding correlation versus causation is so important.
Therefore, it’s important to understand the hidden causal relationships in your data.
Cause and Effect Relationships Rule:
By identifying and analyzing the cause-and-effect relationships between different variables, you can gain a deeper understanding of what drives your product's growth and what factors are most critical to optimize. This helps you make more informed decisions, take the necessary steps to improve your product, and ultimately drive successful growth.
Going out on a limb here… if you understand the cause and effect behind your KPI changes, you can expect your analytics life to be better. The “knowing” you get to with causal analysis is far more fun than the “Guessing” - plus, you can achieve more with less work. You are much more likely to run successful experiments and to take correct action, than compared to running off of the pure correlations that you might have today. And… by being more successful through causal analysis, you will drive a real impact on company growth and gain well-deserved recognition.
An Actionable Analytic Framework:
The causal analyses I’m about to discuss each offer significant value for analytics and growth teams. They provide a framework for identifying the biggest opportunities to improve your KPIs.
Also, each is achievable - you can begin implementing these analyses right away, either manually (read the post here on how to model intent with causal models) or through certain vendor solutions. In this example, the analyses are shown using the Loops platform, but you can learn how to build your own opportunity sizing models from my post on this topic, and in the “Building an Opportunity Sizing Model” section below.
Key info about the sample scenario used in this article:
In the example below, I use a photo/video editing app that offers Free and Paid versions. I will analyze the same features against different KPIs.
Conversion Drivers Analysis
A causal inference model-based analysis on new users, 1st week conversion.
1st week conversion is typical for our example of a photo/video editing app.
Only pre-conversion new user experiences are considered.
The table below shows a list of features that have the highest impact on getting users to convert within the 1st week. The “Split Video” feature has the highest potential to improve the conversion KPI, at 38.63%. What’s very interesting is that if you get all the users to adopt this feature your KPI will go from 21.1% to 38.63%, which represents 82.86% lift, as shown in green on the far right.
The Growth Simulator below allows us to do what-if analysis (sensitivity analysis) to understand if we want to prioritize this opportunity. We want to identify features with high impact and good upside in terms of adoption rate. For example, we see that the Clean Object and Replay features have relatively low adoption compared to others and represent potential growth opportunities.
Retention Drivers Analysis
Free Users
3rd Week Retention
In this causal analysis, I focused on the 3rd Week Retention of free users. Even for free users, improving retention has merit and value - they may convert later, contribute to virality, and provide valuable data for causal analysis, in this case via AI models.
The format of the table and graphic below is the same as shown above for the Conversion KPI, showing the Feature, Adoption Rate, Goal in Adopters, and Maximum Potential impact.
Interestingly, none of the “winners” from the new user conversion analysis above rank at the top of this list of high-impact features for 3rd Week Retention. Instead, the “Audio AI Fix” stands out with a maximum potential lift of 113.26%. Additionally, the features listed in rows 2 and 3 (“IAdd Text” and “Glitch Video Effect”) also show 90%+ maximum potential impact. All three features have low Current Adoption and high Goal in Adopters rates.
Engagement Drivers Analysis
Paying Users
Engagement is defined as the number of times the core value "export video/photo" is performed in a 28-day window.
Unlike the other analysis, this one does not look at new users. It is focused on existing, paying users, previously activated and with a clear pattern of usage.
The analysis looks at patterns of engagement and identifies the impact of adopting different features on the level of engagement (Exporting a Video/Photo over 28 days). The analysis identified multiple features with a positive impact on the Export action. In the table below, you can see the projected KPI lift in the number of “exports” in a 28-day window for key features based on various lifts in the adoption rate (the growth simulator enables you to play with different levels of intent). The “Magic Eraser“ feature potential lift in this engagement KPI is 14.42%, for example. The table is ranking the feature by the potential lift:
It is interesting that two of these features were not included in our top features for our new user conversion analysis, nor on our top features for 3rd week retention.
This analysis highlights the difference that the user’s stage has on the impact of features on a KPI - i.e. the drivers for existing users that have already developed a habit around your product are different than for new users. The insight informs our understanding of the user lifecycle and the decisions we make to optimize our KPIs through each stage. In the table below, “Magic Eraser” for example, can drive a 14.42% lift in the amount of Exports per 28-day window.
Feature Funnel Analysis
While we have found interesting opportunities from the previous analyses, this next step involves figuring out what to do with that information to increase the adoption of these features and journeys.
The best way to look at this is through a Feature Funnel analysis, where the funnel for each feature is analyzed at its final stage. This analysis makes it very clear where new opportunities lie, and where existing efforts have had little result.
The table shows the key four measures of this analysis:
The table and the graphic below highlight the two extreme cases of opportunities in our photo/video editing app example.
Key Insights:
Having presented these different analyses, I’ve demonstrated that you can get to some really deep insights using causal analytics. These are the key insights to keep in mind:
Features Rarely Fit Every Stage: The features that initially attract users to purchase the product are not necessarily the same features that get them to form a habit and engage more with your product later on. You need to tailor the right journeys based on the user’s maturity / stage, and the KPIs you are looking to move.
Go deeper: To understand why users are not adopting certain features, you’ve got to dig deeper. Doing so will make your insight much more actionable.
Optimize Your Free vs Paid Mix: Identify which features should be offered in the Free version versus those that should stay in the Paid version. Ensure you are not giving away capabilities for which users would willingly pay.
Features that drive conversion can be promoted in the paywall, building awareness of them, then maybe offering them free for X days.
Features that drive engagement for paid users should probably be kept in the paid version. Promote them later in the user journey to increase engagement - which leads to lift retention, upsells, virality, etc.
Features that drive free retention but don't add a lot for monetization should be kept in the free version and promoted there.
Acknowledge the Value versus Frequency Trade-Off Examine the feature value/frequency balance. Don’t expect the sexy features that attracted your users to your platform and had high value for new users, to be the features they run most often down the line. By digging deep into your product usage data, you can identify these trends, enabling you to maximize KPIs at every stage of the user lifecycle.
How to Build an Opportunity-Sizing Model
The opportunity sizing model is derived from the causal insights models. While a complex analysis, opportunity modeling can help you discover deep and sometimes unexpected insights. Because you have goals to meet, you need to prove that the potential lift from features/journeys could significantly contribute to your KPIs. For example, growth teams run many initiatives that, even if they achieve their maximum potential, would not affect the numbers significantly. Conversely, other opportunities are much more significant than they would have ever thought. Causal insight helps minimize the risk of going off in the wrong direction.
Here are the key elements of opportunity modeling - a high-level, simplified overview:
The specific reach: How many users will be exposed to the new feature/experience/launch? You can sometimes use a funnel to measure this, just ensure you only include the user segments that are relevant for the feature.
The potential impact on one core metric. Segmentation is crucial here since different segments might react differently to the tested opportunity. Compare users from similar campaigns with similar attributes. Grouping these users enables you to understand behavior better.
The impact on business metrics: For example, the projected impact on revenue? Let’s assume you improve 2nd-week retention —> how would it affect your WAU or Revenue?
Most product analytics solutions are built as visualization tools - dashboards that might show a correlated feature but without any causal certainty. For the analyses reviewed in this article, you’ll want to build or find a solution that provides the estimated impact of each opportunity presented so you can decide on how you will prioritize them.
In Conclusion:
Causal analytics deliver robust insights and quantify the impact of each opportunity. Having these hypotheses, the ones that will win, is a complete paradigm shift for growth and analytics teams. With causal analytics, they can make a real impact on growth.
And when growth happens, people get well-deserved recognition and yes …even promotions!
Thanks, Tom!
Learn more about Loops - You don't have insights; You have dashboards.
Learn more about product analytics - Loops blog.
Thanks for reading, everyone. Until next week!
Subscribe to Data Analysis Journal
Where product, data science, and analytics intersect. Trusted by tens of thousands of data scientists around the world