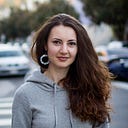

Discover more from Data Analysis Journal
Love And Hate Between Product Management And Analytics - Issue 104
What are the main responsibilities and expectations of a product analyst, and how can you foster a healthy collaboration between product and analytics?
Hello from New York! And welcome to another edition of Data Analysis Journal, a newsletter about data and product analytics. If you’re not a paid subscriber, here’s what you missed this month:
9 Worst Practices In SaaS Metrics - a recap of Christoph Janz’s presentation about common mistakes in SaaS across calculations and approaches for reporting MRR, churn, retention, and more.
Party Like It's 2019: Product Trends Analysis In A Post-2020 World - product trends analysis of user behavior done by Amplitude that illustrates the post-pandemic shift across many domains. Yes, DAU for eCommerce has increased by 60% and holds steady. Good luck with estimating your YoY growth.
Top 10 Tools Every Analyst Should Have - Here, I share my toolkit that saves me time while working on smaller projects that are simple but possibly time-consuming. They can help you to work faster, automate some repetitive tasks, or serve as another source of data validation.
First of all, this month I am excited to announce two more partnerships!
My journal is partnering with the largest Data Conference in Southern California - DCLA! It will be happening in Los Angeles on August 13th-14th at the University of Southern California campus (and also online).
Spearheaded by Subash D’Souza and organized and supported by a community of volunteers, sponsors, and speakers, Data Con LA features the most vibrant gathering of data and technology enthusiasts in Los Angeles. The key speakers include leaders and mentors across data science, ML, AI, data analytics, and more. It also will include special sessions dedicated to NFTs, innovation, and blockchains.
A little history: Data Con LA began as Big Data Camp LA in 2013, with just over 250 attendees and growing significantly every year. It was rebranded to Big Data Day LA in 2014, then to Data Con LA with over 2000 attendees in 2019. In response to the COVID-19 pandemic, DCLA had its first successful virtual conference in 2020 and then in 2021 with over 1000 virtual attendees.
This year, it’s back in-person! Along for the ride is IM Data 2022, who are joining forces to create the largest data science event of the year! There are will be a great lineup of speakers and over 100 sessions across:
AI / ML / Data Science
Data Engineering
Data Infrastructure & Security
Emerging Tech
Data 4 Good
BI / Reporting / Business Use Cases
If you are interested in participating, reach out to me to learn more about how to get involved or get your tickets here. After the conference, I also will be sharing a recap of my favorite sessions and talks.
In addition, this year DCLA also will be running Data Con LA Startup Program & Pitch Competition! DCLA provides early stage (pre-seed and seed stage) projects and companies access to a vast network of technical and engineering subject matter experts, advisors, Angel and Venture Capital Investors, and PR opportunities. If you have a startup idea that you want to pitch, you can fill out this form, but hurry - the deadline is this Friday, July 29. The Global Pitch Competition will take place virtually on Thursday, August 4th.
One more partnership I am excited to announce this month - with Women In Analytics!
WIA is an analytics community whose mission is to increase the visibility of women making an impact in the analytics space, and to provide a platform for women to lead the conversations around the advancements in analytical research, development, and applications.
Last year, I co-authored a book with some fantastic WIA analysts - Analytics Interpreted: A Compilation of Perspectives - a curation of leadership, perspectives, and frameworks across the most relevant topics in analytics, such as data ethics, analysis, strategy, modeling, visualization techniques, case studies, etc. This year, to support WIA I offer a free annual subscription to my newsletter and journal to all WIA members. If you are looking for a mentor, support, or an open and safe community to be part of - WIA is the place.
Product and Analytics united for good
Today I want to address some common questions about product analytics I received from my readers:
What are the main responsibilities of a product analyst?
How much is the actual overlap between analyst and product manager?
How do you foster a healthy collaboration between product and analytics?
Note: I will be using PM as “Product Manager”. In my view, I envision a Product Manager as a Product Owner responsible for the development, rollout, and support of a feature, functionality, or app. Program Manager or Project Manager is a completely different role that I won’t be referencing today.
Product Analytics — the 5W1H Approach to Building Stickier Apps
There are 3 main areas that product analysts are primarily responsible for:
Data reporting and analysis.
Experimentation and rollouts monitoring and analysis.
Analytics approval and governance.
Let's break them down.
1. Data reporting and analysis
This is the heaviest responsibility, which includes metrics definition, calculation, coding, and visualizations. You will need to translate the business definition of metrics and KPIs into SQL and communicate via dashboards and reports. This includes weekly metrics monitoring, monthly KPI reports, impact, opportunity size, and estimation analysis.
From my perspective, the main KPIs definitions should come from PMs, as they know their product best. Analysts help to adjust its definition and formulas based on available data and make it accessible for monitoring and reporting.
2. Experimentation and rollouts monitoring and analysis
I wrote about experimentation A LOT. To summarize, this responsibility includes new feature rollouts, monitoring product adoption, and working with A/B tests. PMs come up with the test idea, hypothesis, and required audience. Analysts are responsible for the following stages of experimentation:
Prep launch - confirm the hypothesis, set the Baseline metrics, suggest MDE, and estimate the test timeline to reach significance and sample size.
Test launch - closely monitor A/A test, validate traffic dissemination, and QA data to validate the right audience.
Test monitoring - monitor the test flow.
Test analysis - once data is significant and you reach a sample size, analyze the test performance.
Recommendation - communicate to the stakeholder both the test outcome and your recommendation on the next steps (accept variant, close the test, re-launch the test to a different audience, etc)
In some cases after the successful test (when Variant is a winner) you might have to conduct Pre/Post analysis to confirm the test impact and the actual lift in metrics.
3. Analytics approval and governance
Every launch of a new feature or experience requires creating new events to support analytics. Analysts should monitor the creation of new events and properties, making sure they are in line with expected data volume and drive data governance. This responsibility will be different at various companies, as this process is often divided between multiple teams - engineering, product, and analytics. The most common scenario:
PMs create a list of events they wish to have in order to monitor CTAs, CVRs, and user behavior. They pass it to analytics.
Analysts are responsible for making sure they will answer the questions, will be sufficient for analytics, and follow the set format and practice. Once new events are approved, pass them back to the PM to send for development.
The development/engineering team creates new events and new properties, and the QA team works on validating them.
So where is the culprit?
I keep hearing stories about tensions between product managers and analysts at many companies. I personally also have a few examples of misalignment, stepping on each other's toes, or miscommunication. Naturally, there is a lot of overlap between these 2 roles, and given how data becomes more accessible, better specialization and synchronization are vital.
As a true self-respected analyst, I do think that analysts are the most important and impactful role of all. They are a guiding shining star in the dark ocean, responsible for bringing a ship to the light. I also believe that every product analyst can be a great product owner, but not every product person can be an analyst.
Jokes aside, data democratization requires more collaboration and alignment between these domains. Here are some examples of common culprits:
using different data sources for reporting
misinterpreting experiment results
ignoring the test procedure, checks, rushing with rollouts, testing all the things
over-estimating the impact of product initiatives
misreading or not understanding metrics definitions
confusing and complex dashboards and reports
Once teams are allied on the right data sources, current reporting limitations, architecture challenges, and experimentation complexity, things usually get better. Getting there will require investing in documentation (from both sides), weekly check-ins, friendly fast-accessible dashboards, and hot chocolate retros.
It’s a holy union of sorts - when product and analytics are merged into one force, connected via the work streams and OKRs, great things happen!
Thanks for reading, everyone. Until next Wednesday!
Subscribe to Data Analysis Journal
Where product, data science, and analytics intersect. Trusted by tens of thousands of data scientists around the world